Seminar: Efficient Motif Discovery in Large-Scale Time Series Data.
- Speaker: Dr. Jessica Lin
- Computer Science Department, George Mason University
- Date: Friday, March 22, 2019
- Time: 1:00pm - 2:00pm
- Location: Room T3 (NVC)
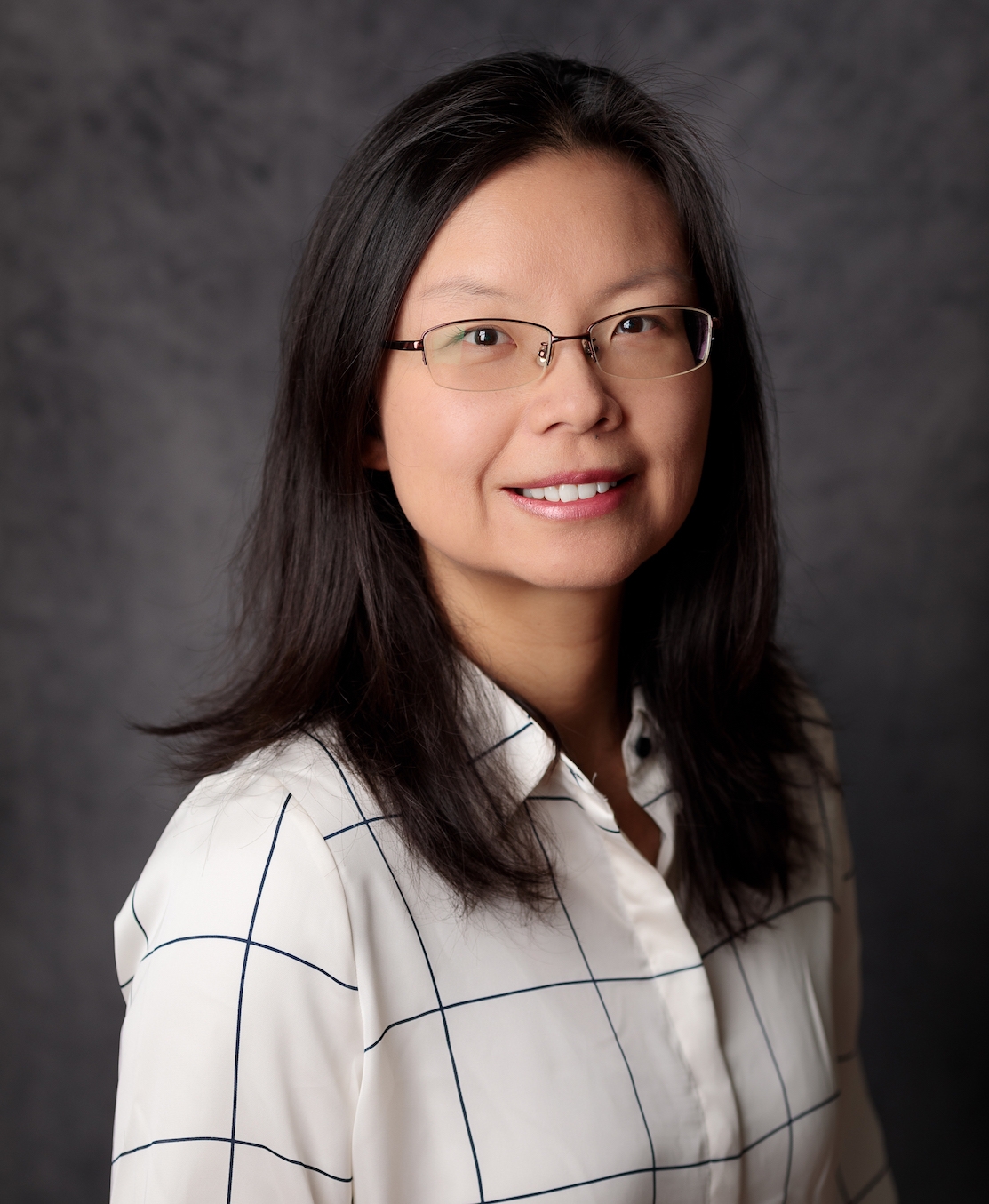
Abstract
Massive amounts of data are generated daily at a rapid rate. As a result, the world is faced with unprecedented challenges and opportunities on managing the ever-growing data, and much of the world's supply of data is in the form of time series. Time series data mining has thus attracted an enormous amount of attention from researchers and practitioners in the past two decades. This presentation will focus on a particular pattern discovery task called time series motif discovery-- the discovery of repetitive similar patterns that occur in time series data. In this talk, I will discuss the common challenges of time series motif discovery and present our recent work in this problem domain. I will demonstrate the utilities of motif discovery in various data mining tasks such as association rule mining, classification, and anomaly detection.
Speaker's Biography
Dr. Jessica Lin is an Associate Professor in the Department of Computer Science at George Mason University. She received her PhD from University of California, Riverside in 2005. Dr. Lin's research interests focus on the mining of massive time series and spatiotemporal databases. More specifically, she has published work on anomaly detection, frequent pattern (motif) discovery, contrast set and rule mining, clustering, and visualization on time series and spatiotemporal data. She has worked on several collaborative projects in diverse disciplines including medicine, activity recognition, CPU manufacturing, finance, geoinformatics and meteorology, with support from the National Science Foundation (NSF), Sandia National Laboratories/Department of Energy (DOE), U.S. Army, Naval Research Lab (NRL), and Intel Corporation. She is an Associate Editor for the Pattern Recognition Journal. Dr. Lin has served as a PC member in top-tier data mining conferences including KDD, SDM, ICDM, ECML/PKDD, and CIKM.