Seminar: Robust Machine Learning.
- Speaker: Dr. Ananthram Swami
- Army Research Lab
- Date: Friday, Oct 25, 2019
- Time: 1:00pm - 2:00pm
- Location: Room 214 (NVC)
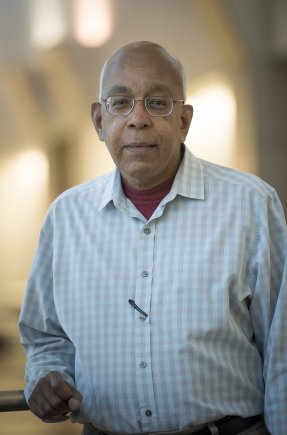
Abstract
Modern machine learning systems are susceptible to adversarial examples; inputs that preserve the characteristic semantics of a given class, but whose classification is incorrect. Current approaches to defense against adversarial attacks rely on modifications to the input (e.g. quantization) or to the learned model parameters (e.g. via adversarial training), but are not always successful. We discuss some of the enablers of successful adversarial attacks via an empirical analysis of commonly used datasets. We propose a novel defense mechanism in which the model outputs are represented and decoded in a fundamentally different way from current approaches. We demonstrate improved robustness via detailed testing on commonly used datasets. The resulting architecture has several advantages: it yields meaningful probability estimates, it declares uncertainty when it should, is fast during training and testing. Time permitting, the talk will include a discussion of novel approaches to detection of adversarial examples.
Biography
Dr. Ananthram Swami is with the US Army CCDC Army Research Laboratory and is the Army's Senior Research Scientist (ST) for Network Science. Prior to joining ARL, he held positions with Unocal Corporation, USC, CS-3 and Malgudi Systems. He was a Statistical Consultant to the California Lottery, developed a Matlab-based toolbox for non-Gaussian signal processing. He has held visiting faculty positions at INP, Toulouse, and currently at Imperial College. He received the B.Tech. degree from IIT-Bombay; the M.S. degree from Rice University, and the Ph.D. degree from the University of Southern California (USC), all in Electrical Engineering. Swami's work is in the broad area of network science, and more recently in the area of adversarial machine learning. He is an ARL Fellow and a Fellow of the IEEE.